Whether IT leaders opt for the precision of a Knowledge Graph or the efficiency of a Vector DB, the goal remains clear—to harness the power of RAG systems and drive innovation, productivity, and enhanced user experiences.
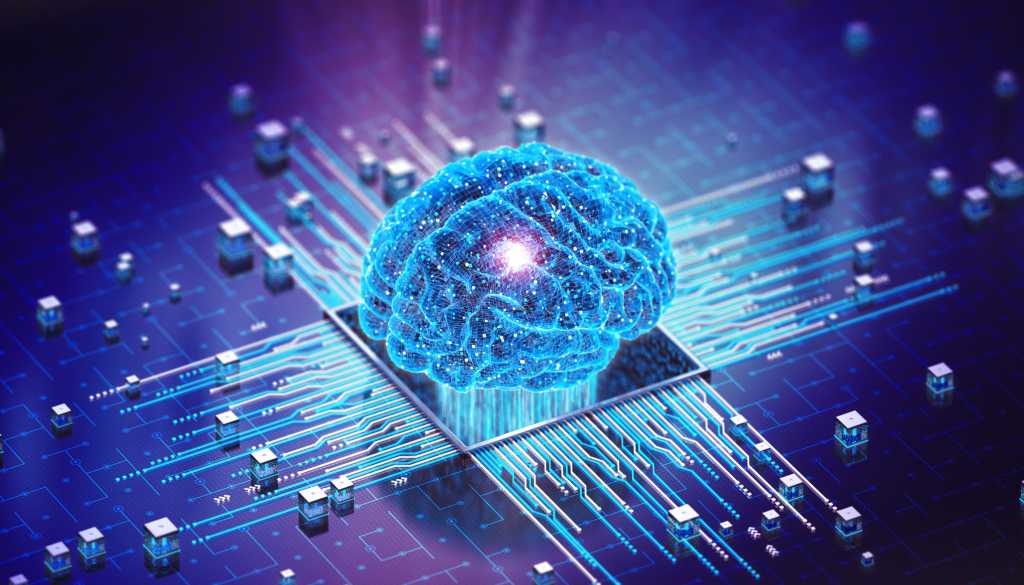
Generative AI (GenAI) continues to amaze users with its ability to synthesize vast amounts of information to produce near-instant outputs. While it’s those outputs that get all of the attention, the real magic is happening behind the scenes where complex data organization and retrieval techniques are allowing these connections between disparate data points to be made. It is also the area where many technologists differ on the best approach.
At the heart of the issue is retrieval-augmented generation (RAG), a natural language processing technique combining data retrieval with a GenAI model. With RAG, for the first time, GenAI-powered solutions can enhance their own knowledge and content generation by retrieving information from external sources, instead of just relying on pre-programmed data sets. This monumental leap forward has wide-ranging implications for business, society, and technology. But the critical step of data preparation can’t be overlooked — and today, it uses decades-old technologies.
Choosing the right data architecture
Currently, there are two primary technologies that are used to organize the data and the context needed for a RAG framework to generate accurate, relevant responses: Vector Databases (DBs) and Knowledge Graphs. While these data management technologies may not be as exciting as RAG, if CIOs want their shiny new toys to work properly, Vector DBs and Knowledge Graphs need to be a top priority.
The challenge is: both involve very different executions and – at some point – CIOs will need to make the call on whether it would be better to use a Vector DB or a Knowledge Graph. Which one is best? It depends.
Before moving forward, CIOs consider the problem they are trying to solve with RAG and how complex their data is, then compare their needs with each data architecture’s pros and cons.
A Vector DB stores and manages unstructured data — text, images, audio, etc. — as vector embeddings (numerical format). These embeddings capture the semantic relationships between the data points. When the RAG framework searches Vector DB to retrieve data, it quickly looks for mathematically close vectors, which imply similar meaning, not just keyword matching.
Knowledge Graphs, by contrast, represent data as a network of nodes (entities) and edges (relationships). They can handle more complex, nuanced queries based on the types of connections, the nature of their nodes, structure, and properties. They can also capture rich semantic relationships that might be lost in a vectorized embedded space.
As a result, it is best to choose a Knowledge Graph when the organization needs a powerful tool for structuring complex data in an interconnected network that facilitates data representation and traces the relationships and lineage between the data points. Knowledge Graphs are handy where understanding the context and connections within the data is essential. The LLM can say, ‘My answer came from these triples or this subgraph.'”
Reasons to choose a Vector DB over a Knowledge Graph include lower cost and speed. The Knowledge Graph can be expensive, but if the use case calls for a Knowledge Graph — where the information is needed in a way that only a Knowledge Graph can provide — then the price is worth the accuracy of the output.
When to choose Knowledge Graphs vs. Vector DBs
Specific use cases where Vector DBs excel are in RAG systems designed to assist customer service representatives. These employees are often tasked with answering a wide array of customer queries, ranging from procedural questions like changing coverage on an existing policy to more complex inquiries such as filing an auto insurance claim. In these scenarios, the RAG system leverages a Vector DB to dynamically fetch the most relevant answers from a structured Standard Operating Procedures knowledge base. This improves customer satisfaction by reducing wait times and ensuring that customers receive consistent information.
Vector DBs perform so well in these contexts because they can perform semantic searches. They transform text queries and documents containing potential answers into high-dimensional vector spaces, facilitating the identification of content whose semantic content most closely aligns with the query.
Knowledge Graphs tend to perform well in areas like complex insurance claims adjustment, where adjusters must navigate through a labyrinth of interconnected data points. This role demands not just the retrieval of information but a deep understanding of the relationships and interdependencies among various entities. Knowledge Graphs shine in this complex environment by providing a structured representation of relationships between entities, such as policies, claims, and customers.
As organizations navigate the complexities of implementing RAG, choosing between Vector DBs and Knowledge Graphs becomes pivotal. While both offer unique advantages, understanding the specific data needs and the intricacies of a particular use case is paramount. Whether CIOs opt for the precision of a Knowledge Graph or the efficiency of a Vector DB, the goal remains clear: to harness the power of RAG systems and drive innovation, productivity, and enhanced user experiences. Choose wisely and embark on a journey where the convergence of human ingenuity and machine intelligence redefines the possibilities of collaborative problem-solving in the digital age.
Learn more about how EXL can put generative AI to work for your business here.
About the author:
Anand Logani is the chief digital officer at EXL, a leading service provider of data- and AI-led analytics, operations, and solutions.